HAA4D Dataset
HAA4D is a challenging human action recognition 3D+T dataset that is built on top of the HAA500 dataset. HAA500 is a curated video dataset consisting of atomic human action video clips, which provides diversified poseswith variation and examples closer to real-life activities. Similar to Kinetics, the original dataset provides solely in-the-wild RGB videos. However, instead of using existing 2D joints prediction networks, which often produce inaccurate results when joints are hidden or not present, HAA4D consists of hand-labeled 2D human skeletons position. These accurate features can not only increase theprecision of the 3D ground-truth skeleton but also benefit training for new joints prediction models that can reasonably hallucinate out-of-frame joints. The labeled 2D joints will then be raised to 3D using EvoSkeleton. HAA4D is thus named with its accurate per-frame 3D spatial andtemporal skeletons for human atomic actions. The dataset can be divided into two categories: primary classes and additional classes. Primary classes have twenty samples per class, while additional classes contain two examples per class.
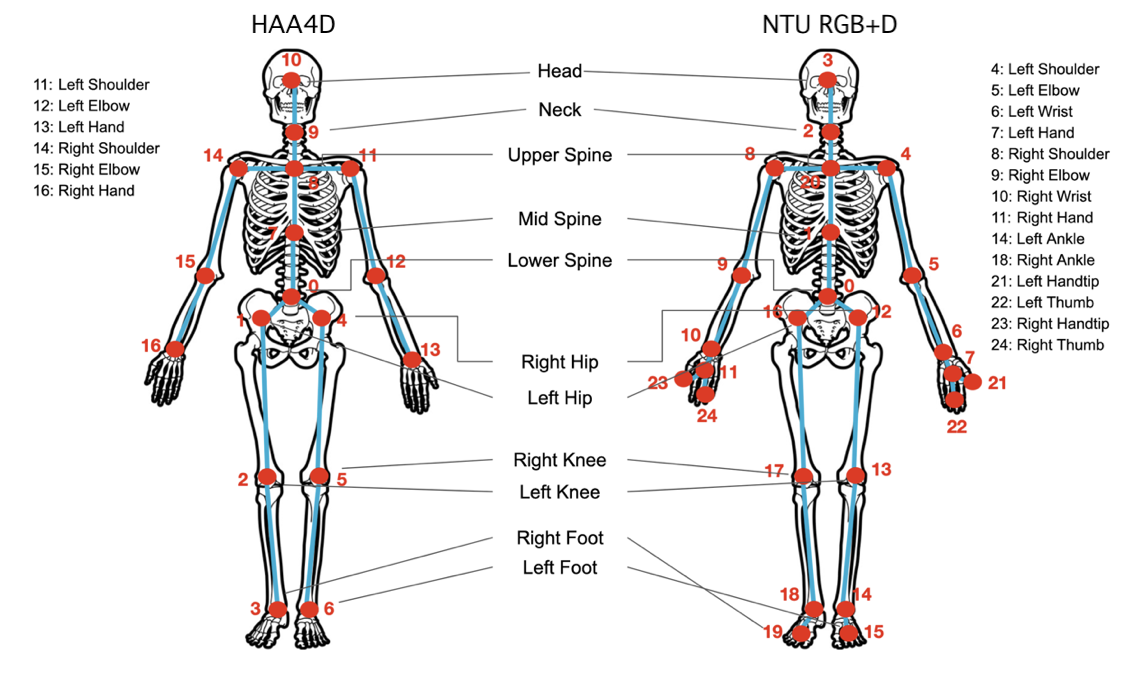
1. Action Classes
1.1 Primary Classes (155)
1.2 Additional Classes (145)
1.3 Globally Aligned Skeletons
HAA4D (40)
NTU RGB+D (40)
2. Dataset Details
2.1 Comparison with other pubic datasets
2.2 Dataset Summary
Classes | Total Samples | Globally Aligned Samples | Total Frames | Min Frames | Max Frames | Average Frames | 2 Examples | 20 Examples | Person per Example |
300 | 3390 | 400 | 212042 | 7 | 757 | 63 | 145 classes | 155 classes | 1 |
2.3 Evaluation Benchmark
For actions with 20 examples per class, video indexes 0 to 9 are used for training, while videos from 10 to 19 are used for testing. We perform data augmentation on the first ten samples, and among all, videos 8 and 9 are used for validation. For actions that contain only two samples, the one with a smaller index serves as the query, and the other serves as the support.
3. Examples
Here we provide some examples in our HAA4D dataset. The image on the left shows the 8-frame sampling from different actions. We use our global alignment model (GAM) to rectify the input skeletons and bring them to the same coordinate system that all actions start at facing the negative z-direction. Some samples of globally aligned skeletons can be seen on the right. More samples can be access in here.
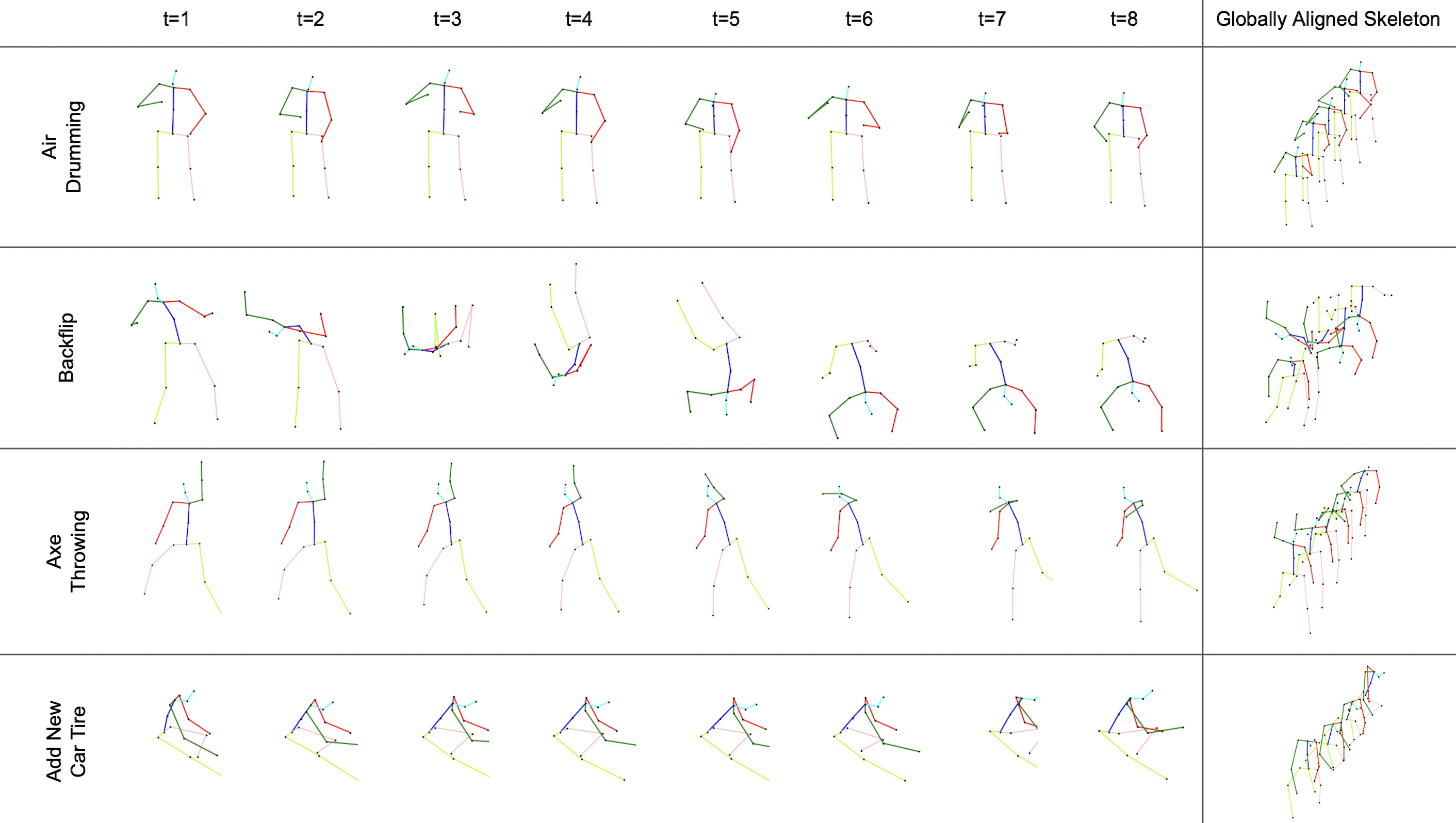
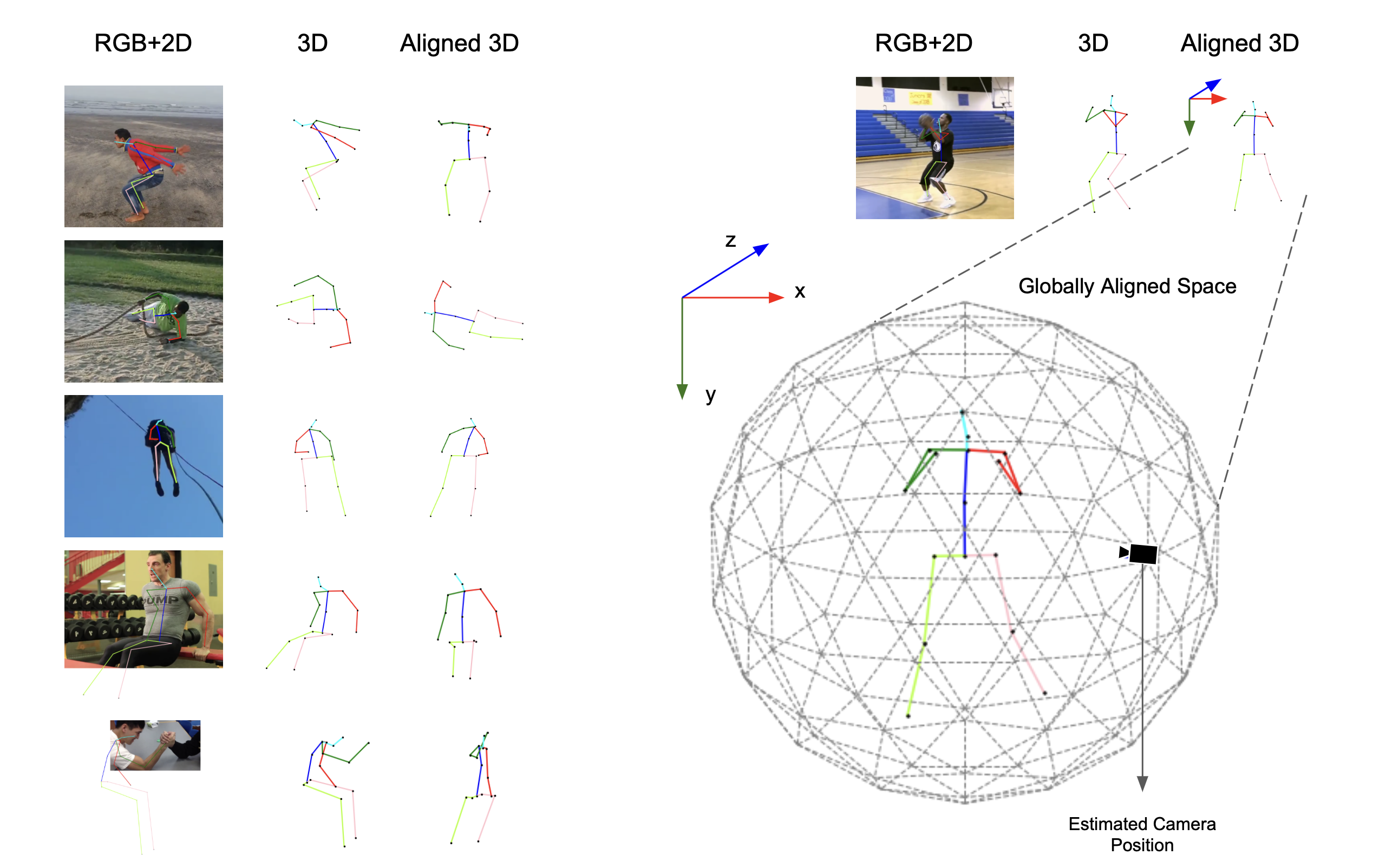