Alumni Yuan Yuan and Hao Wang Created an Artificial Intelligence Model to Identify Parkinson's Disease
Yuan Yuan works as a Postdoc Associate at MIT's Computer Science & Artificial Intelligence Lab (CSAIL) alongside Dina Katabi. Prior to MIT, she got her Ph.D. at HKUST, where she was guided by Prof Dit-Yan Yeung. She was a visiting research scholar at Carnegie Mellon University's Robotics Institute. Her research interests include machine learning, computer vision, artificial intelligence for healthcare, and medical AI. Her work has featured in prestigious journals and conferences.
Hao Wang is an Assistant Professor at Rutgers University's Department of Computer Science. He was formerly a Postdoctoral Associate at MIT's Computer Science & Artificial Intelligence Lab (CSAIL), where he collaborated with Dina Katabi and Tommi Jaakkola. In 2017, he was the lone recipient of the School of Engineering PhD Research Excellence Award and obtained his PhD from the Hong Kong University of Science and Technology.
Yingcong Chen is an Assistant Professor at HKUST(GZ) AI Thrust and an Affiliated Assistant Professor at HKUST Department of Computer Science & Engineering. Prior to that, he worked as a Postdoctoral Associate at the Massachusetts Institute of Technology's Computer Science and Artificial Intelligence Lab.
Three of them, together with other academics, recently published a paper titled "Artificial Intelligence-Enabled Detection and Assessment of Parkinson's Disease Using Nocturnal Breathing Signals." This paper has received over a hundred new stories from 83 different publications, including MIT News, Forbes, The Washington Post, Fierce Biotech, and others. They realise that Parkinson's disease is famously difficult to diagnose since it is based mostly on the occurrence of motor symptoms such as tremors, stiffness, and slowness, yet these symptoms sometimes arise years after the illness has begun.
They created an artificial intelligence (AI) model to identify Parkinson's disease (PD) and follow its development using nocturnal breathing patterns. The model was tested on a large dataset over 7 thousands people, which included data from many hospitals in the United States as well as multiple public databases.
Furthermore, the model can assess Parkinson's disease in a touchless method in the home environment by collecting respiration from radio waves that bounce off a person's body during sleep. Their research illustrates the possibility of objective, noninvasive, at-home evaluation of Parkinson's disease, as well as preliminary indications that this AI model may be beneficial for risk assessment prior to clinical diagnosis.
They mention that the first challenge is gathering a large dataset. The data for this study was collected during a two-year period in partnership with the University of Rochester, Mayo Clinic, and Massachusetts General Hospital. The second problem is to solve the dataset's machine learning concerns, such as data and data comes from different institutions and devices.
This paper has a significant impact on the study of Parkinson's and the direction of medical AI. "We've had no therapeutic breakthroughs this century, suggesting that our current approaches to evaluating new treatments is suboptimal," says Ray Dorsey, a University of Rochester professor of neurology and Parkinson's specialist who co-authored the article. Dorsey adds that the study is expected to be one of the largest sleep studies on Parkinson's ever performed.
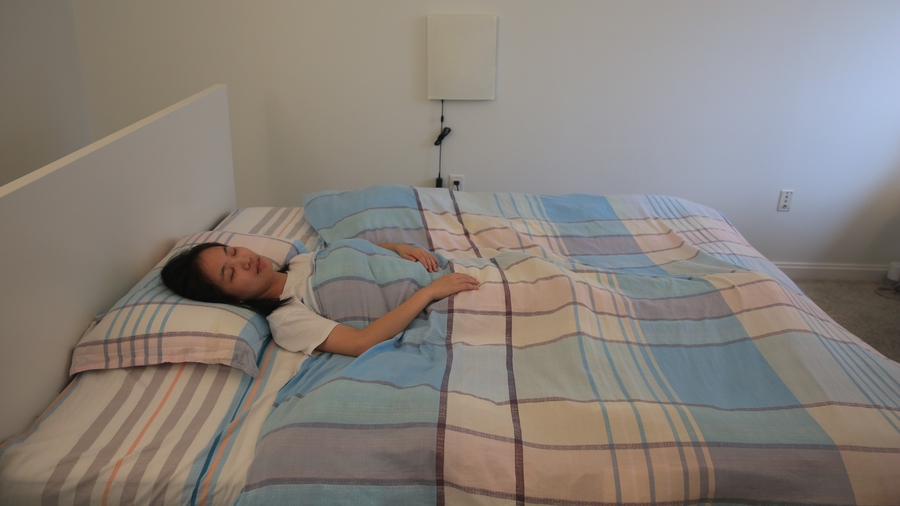
Paper device figure
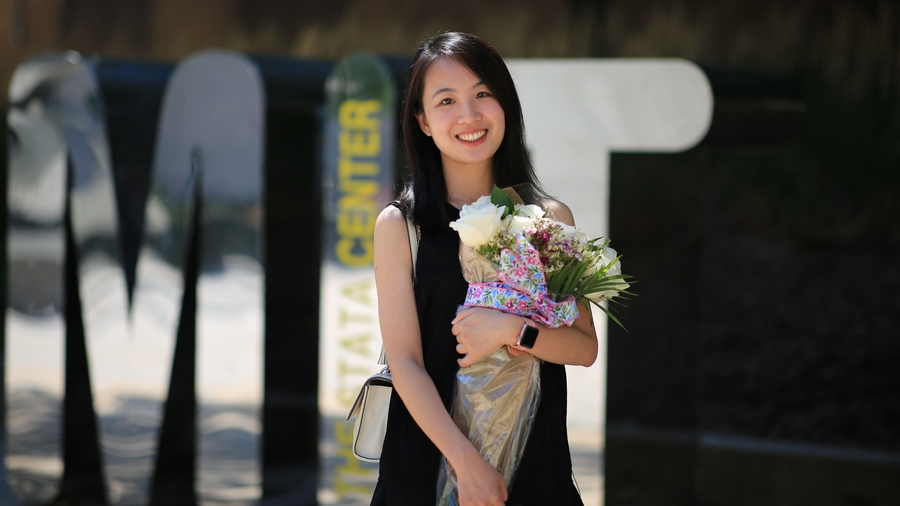
Photo of Yuan Yuan

Photo of Hao Wang